This robotic limb merges machine learning and manual control
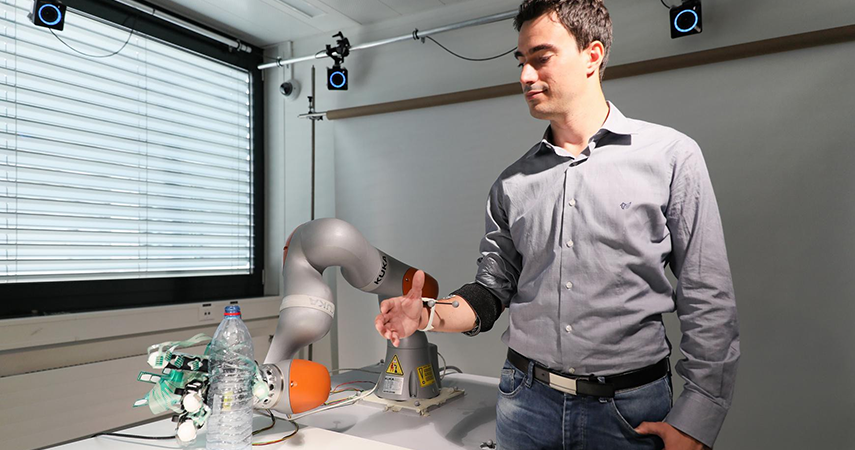
Image Credit: EPFL / Alain Herzog
Scientists from École polytechnique fédérale de Lausanne (EPFL) have developed a new approach for operating a robotic limb using machine learning and manual control.
The research and development of neuroprosthetics is getting better every day. New breakthroughs in this nascent field are now successfully being demonstrated. As our search for symbiosis between humans and robots continues, scientists from the EPFL have broken new ground by developing something entirely novel, adding automation to a manually controlled robotic limb.
The development by EPFL combines individual finger control and automation to allow the prosthetic limb to grasp and manipulate objects in ways not seen before in robotic hand control, successfully merging the two fields of neuroengineering and robotics.
Previous attempts at neuroprosthetics have often focused on end-to-end control of a limb using neural transmitters. The robot copies the user’s exact signals from the start of an action to its conclusion. Moving the hand towards the object, opening the grip, closing the grip around the object, and performing the desired movement. However, this development imparts some autonomy to the arm by using sensors and machine learning algorithms.
In the demonstration, the user retrieves an object – in this case, a bottle – with a robotic limb, directing the hand towards the object. Then, instead of each human finger being recognised and translated to the robotic arm, it instead focuses on adjusting its own grip and finger placement, finding the best place to handle the object and adjusting in realtime to slips and subtle movements.
Aude Billard, Learning Algorithms and Systems Laboratory lead at EPFL said: “When you hold an object in your hand, and it starts to slip, you only have a couple of milliseconds to react…The robotic hand has the ability to react within 400 milliseconds. Equipped with pressure sensors all along the fingers, it can react and stabilize the object before the brain can actually perceive that the object is slipping.”
A new approach to robotic prosthetics
To achieve this method of shared control, the user must first train an algorithm with their own movements, building a dataset of intentions and tailoring the robotic limb to their unique muscular activity patterns. The scientists then set about engineering the algorithm to initiate automation when the user attempts to grip an object.
The algorithm filters through the noisy muscle signals to decode and interpret the user’s exact intention based on the trained dataset. It then translates this to finger movements on the prosthetic hand, which closes its grasp when the sensors have successfully identified the target object.
Without using visual signals, this tactile method affords the prosthetic hand the ability to conclude the size and shape of objects, autonomously acting on that information.
Silvestro Micera, EPFL’s Bertarelli Foundation Chair in Translational Neuroengineering said: “Our shared approach to control robotic hands could be used in several neuroprosthetic applications such as bionic hand prostheses and brain-to-machine interfaces, increasing the clinical impact and usability of these devices.”
This new method could be applied to neuroprosthetics for amputees who might not have the ability for individual finger control. Rather than reading muscle signals on an amputated limb, the prosthetic limb takes over, allowing for a robust and accurate grip based on intention rather than direct signals.
Pioneering work in robotic limbs by the likes of EPFL, alongside many others in the field, are propelling this rapidly advancing technology to new heights. Soon, we could see neuroprosthetics ready for use in daily life, both near and remote, in a number of applications, benefitting the lives of millions.