NLP bias and its impact on AI
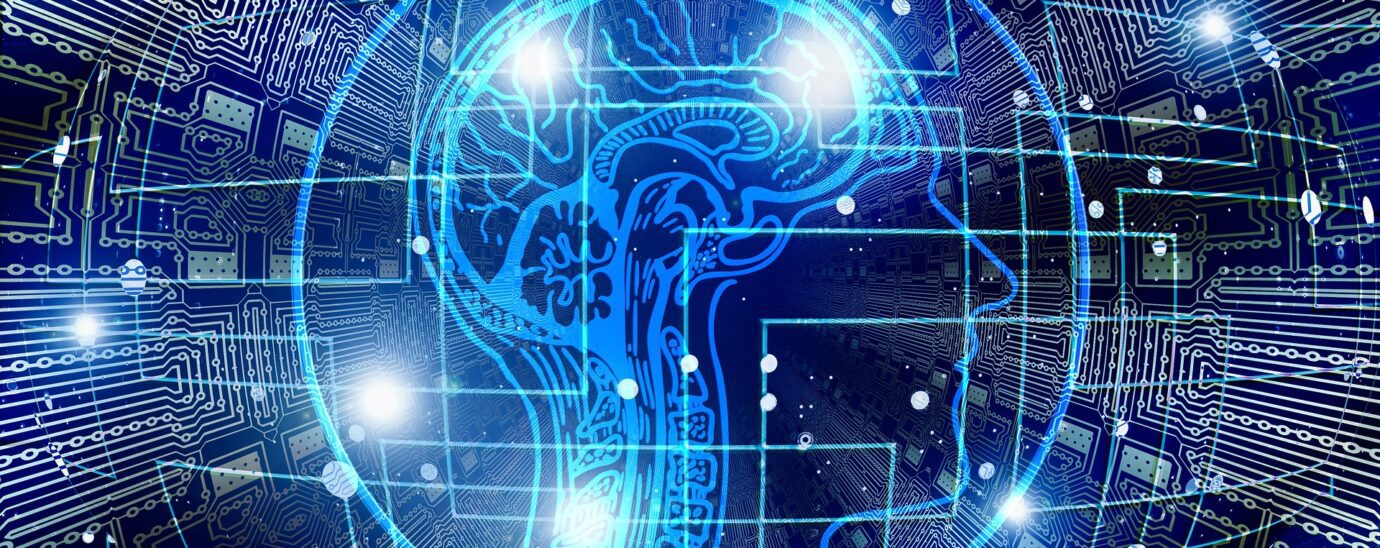
Natural Language Processing (NLP) can be divided into two broad areas: Natural Language Understanding (NLU) and Natural Language Generation (NLG). NLU is concerned with the use of computers to understand the semantic relationships between words in natural language texts, while NLG is concerned with the generation of texts that mimic the semantic complexity of natural language texts.
These tools can be applied to various real-world business problems, such as document classification and summarization, named entity extraction, machine translation, fact checking, and question answering. They can help increase efficiency by reducing search time and effectiveness by improving relevance. NLP can be a highly efficient way to use computers to solve problems that traditionally could only be handled by humans.
NLP and speech recognition software
NLP can even assist with Automatic Speech Recognition (ASR). Since ASR aims to process natural language, it can also be understood as part of the NLP category that combines NLU (utterance comprehension) and NLG (generation of natural language output as a transcription of spoken input).
If an explicit distinction is to be made, then NLP can help improve the accuracy of the acoustic model of an ASR system. In this case, a language model (LM) can be used to estimate the probability of a particular syllable or word sequence. This can help, for example, to distinguish homophones, i.e. words that are pronounced the same but carry a different meaning.
Modern LMs can use the context words to estimate overall probabilities. However, recent publications show that the most accurate ASR systems address the problem end-to-end, i.e., the acoustic model is intertwined with the LM and the speech generation model. This makes it increasingly difficult to distinguish ASR from NLP.
Issues of bias in NLP and speech recognition
But there are instances of bias occurring in NLP and ASR that have the potential to derail the use of these technologies. Implementing AI with modern machine learning (ML) involves two main components: an ML model with a specific architecture and a dataset that models one or more specific tasks. Both of these parts can introduce biases.
The black-box nature of ML models can make it difficult to explain the decisions made by the models. Furthermore, models can overfit datasets or become overconfident and do not generalize well to unseen examples. However, in a majority of cases, the dataset used for training and evaluation is the culprit for introducing bias.
A dataset may contain inherently biased information, such as an unbalanced number of entities. Datasets that have been manually annotated by human annotators are particularly prone to bias, even if the annotators have been very carefully selected and have diverse backgrounds. Large corpora obtained unsupervised from the World Wide Web still exhibit biases, e.g., due to differences in Internet availability around the world or differences in the frequency of speakers of certain languages.
The implications of NLP bias
The downside is that populations that are underrepresented in particular data sets are, at best, unable to use an AI system to help them solve the desired task and, at worst, discriminated against because of how the AI predicts outcomes.
Discrimination based on the unfairness of an artificial model becomes a serious problem once AI systems are used to make potentially important decisions automatically and with limited human oversight. In addition, these problems also hinder the progress and acceptance of AI due to the justified mistrust that is generated. As a result, these technologies are most effective when they are used to augment, rather than replace, human input and expertise.
Overcoming and regulating bias in NLP technology
Unfortunately, there is no silver bullet to solve the problem of bias in NLP, ML, or AI in general. Instead, an important component is awareness of the problem and an ongoing commitment to developing AI solutions that improve fairness.
Technically, there are a variety of theories and methods that are being actively researched and developed to improve fairness and explainability. These include but are not limited to measurement and reduction of bias in datasets, principles for balanced training of models, strategies for dealing with inherent uncertainty during inference, and ongoing monitoring of AI decision-making.
The role of ethics
The recent field of Ethics in AI also plays a role in addressing NLP bias. The challenge is that AI is still a relatively young and fast-moving field of research and application. Although it has existed for many years, it is only recently that the deployment has become more widespread. We have not yet reached the plateau of stability, which is required to formulate and codify behaviors and norms, ensuring a fair playing field.
Squirro’s approach to this is threefold, and one that could go a long way if followed by the wider industry: A) ongoing consciousness-raising internally and with customers and prospects around the issue of bias in AI modeling and AI-supported decision making. B) calling for and contributing to industry and government working groups establishing the regulatory framework to operate AI responsibly and C) implementing – not just discussing them – A & B.
NLP is an impactful technology, with a variety of use cases that help businesses be more efficient and effective. It is so useful that the industry cannot afford to let its use be negatively affected by issues of bias. Such technologies work most effectively when they are used to augment human input and intelligence, not replace them. In addition to the above, addressing bias requires focus and industry-wide commitment to mitigate its negative impact.