Embracing the value of AI for payments
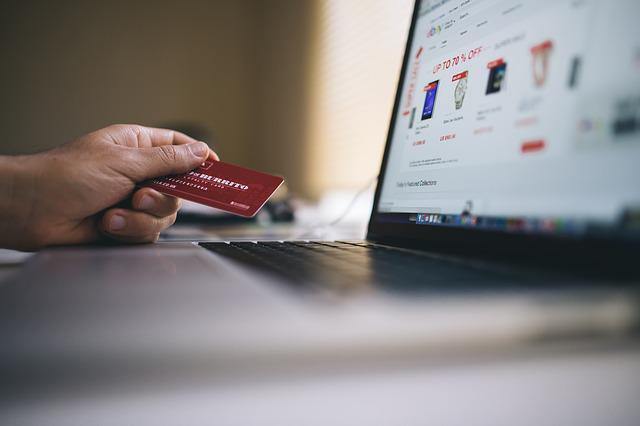
The statistics around digital payments don’t lie. Driven by restrictions on cash and in-person banking during the pandemic, experts are predicting that the global total transaction value in the Digital Payments segment in 2022 could reach more than $8.5bn USD. Even as the world opens up more, this growth is not expected to slow. The same forecast suggests an annual transaction value growth rate to 2026 of 12.76%, totalling a projected amount of over $13.8bn USD in the next four years. eCommerce, the largest segment of this payments volume, is expected to grow to a projected total transaction value upwards of $5.45bn USD in 2022.
While eCommerce’s popularity has presented growth opportunities for businesses, the increase in digital payments has also created a new avenue for fraudulent activity. According to Juniper Research, losses due to online payment fraud will exceed $206 billion between 2021 and 2025. This figure alone, which is nearly ten times Amazon’s net income in the 2020 fiscal year, demonstrates why businesses must prioritise combating fraud. A way to achieve this could be through the use of artificial intelligence (AI) and machine learning (ML) based fraud prevention platforms.
Leveraging intelligent solutions such as AI can significantly reduce fraudulent payments as a result of its ability to analyse a wealth of data and provide recognisable patterns and actionable insights.
The benefits of implementing AI to detect fraud
Although many financial institutions are becoming predominantly digital, some processes heavily rely on manual operations that can be labour-intensive and pose a risk of mistakes due to human error. Additionally, humans need to work shifts which can add up to substantial costs for businesses, while digital payments can happen 24 hours, seven days a week, 365 days of the year. By automating these workflows, AI can help to optimise payment procedures while reducing errors and saving time and resources.
This is primarily achieved through ML, which is a subset of AI that learns from repeat experiments to improve overall performance. ML does this by adapting a particular mathematical function of data using a set of predefined rules and criteria that a payment service provider (PSP) would typically implement across various onboarding platforms. It can then be used to strengthen the security of payments while increasing the efficiency of payment fraud teams. Some examples include:
1. Finding anomalies in large-scale data sets
To go through the sheer amount of data produced by digital payments even for a dedicated team is a mammoth task. For ML though, the more data it can process, the more effective it becomes. By training itself through analysing data sets, ML becomes more accurate, meaning its predictive value increases.
This is especially true in the detection of payment fraud, where ML algorithms can detect what differentiates legitimate and fraudulent transactions from a contextual intelligence standpoint. The data network includes a wide range of metrics including transaction complexities, verticals, and geographies. These insights enable machine learning models to be properly trained to predict risk accurately.
2. Improving operational efficiency
AI isn’t affected by human bias and can work continuously. If programmed well through extensive ML in conjunction with robotic process automation (RPA), payment systems allow for significant reductions in error rates without having to increase complexity or cost. Further, ML in tandem with other critical elements such as advanced risk management rules, tools and processes can work around the clock, meaning businesses can continue to have customers’ transactions processed constantly, without incurring extra costs for having humans working on processing transactions during unconventional working hours.
As a result, businesses can spend less time worrying about the logistics of taking payments and instead focus on growing their business – something that is especially important when trying to reach a worldwide customer base.
3. Prioritising further investigations
While AI doesn’t have human bias, it cannot replace a human workforce completely. There will be instances where human logic should override AI’s black and white decision making.
However, where AI can play a big role is in identifying where an employee’s time should be spent on investigating a potential fraud. To look into an issue can be costly and time consuming, so AI’s data analysing capabilities can be a significant driver to helping prioritise what should be given attention. It can help identify anomalies to eliminate fraud, solve human error, automate repeatable tasks, and help reduce time and cost.
By analysing the elements of historical fraudulent payments, ML can flag newly processed transactions that combine the same or similar elements. If something unconventional is identified, it will send an alert to the fraud team for further investigation. The transaction will then be confirmed or rejected by these experts, giving them the tools to quickly spot and block fraudulent activity.
Innovation growing at the same pace as spending
For good reason, the payment industry is embracing AI to process the ever-growing value of digital transactions. AI offers businesses the opportunity to cut payment processing times, handle massive amounts of data, while also reducing the amount invested in legacy processes. It promises efficiency while dealing with predicted future increases in payment volumes.
Automation and improvement are no longer a ‘nice-to-have’ for businesses. AI provides a solution to operational efficiency and businesses must be sure to innovate alongside the technologies available to the payments sector.